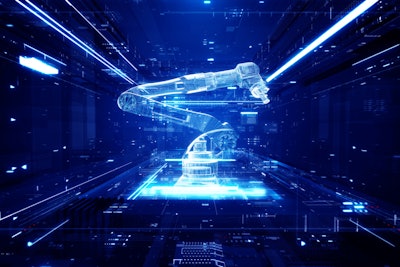
Artificial Intelligence is the latest and potentially most game-changing technology to enter the manufacturing world in decades. Many in the packaging and processing sector have used AI for years, perhaps not recognizing that it is already part of some machines' everyday use. OEM held a Harnessing the Power of AI webinar with David McGraw, Senior Director of Alvarez and Marsal, to discuss this topic, how AI is already utilized in manufacturing, and where it could be applied in the future. To view an On Demand recording of the webinar, click here.
Below is a condensed snapshot transcript of the discussion.
Sean Riley:
I was reading recently in Axios that the United States is one of the slowest adopters and least positive about AI as beneficial to the workplace. Why do you think Americans, in general, are hesitant to embrace AI, particularly in the workplace?
David McGraw:
It's a question I've thought about a lot. There's a handful of reasons. One of them might be a little fear. But that's not as big as people are used to doing the work the way they have always done it. They find themselves to be—or at least their perception of their own work—very productive. Some people may feel that they have limited bandwidth to work a new system or software like AI into their daily workflow. I think that's just as big as anything else.
On top of that, AI is still kind of a black box for many people at the end of the day, so that also turns off some people.
Sean Riley:
Do you believe people fear that AI will take their job?
David McGraw:
That question gets a little more complicated to answer every day. My point of view, at least in the short term, is I don't think AI will take your job. But folks who embrace AI and make it part of their daily workflow are much better positioned to take your job.
The example I give is if you had someone who successfully used AI in their workflow and became two, three, four, or even ten times more efficient at their job, obviously, a company wouldn't need as many people to do that job. It's really the collaboration between human and AI.
Sean Riley:
You liken the current state of AI to when the Internet came on the scene.
David McGraw:
I can even give you the analogy I like to use. So maybe I'll start with the analogy, and we can peel it back. My first job out of college was as a programmer. I remember sitting next to folks who were older than me, and they had their way of doing things.
If you're a technologist, you never write perfect code, not right out of the gate. You're fixing many bugs and researching to better understand the issue. I would use the Internet because, just coming out of college, the Internet was starting to become popular, and there was generally good information.
I would electronically search to find an answer or open up a PDF of the reference manuals and search across it. But the folks I work with would always laugh as they bring in a wheel barrel of 10,000-page reference manuals and say, “All your answers are right here.” The point is that it was far more efficient to utilize the Internet. Within a few years, I didn't see those folks anymore; they had disappeared from the job and potentially from the workforce altogether because they weren't willing to adapt to new technology.
I see AI as being more transformational. With the internet, it took about seven or so years, probably longer, to monetize it. Then we moved to mobile apps, and it was really COVID that pushed mobile apps to the forefront and gave a lot of opportunities to monetize. Now, here comes AI, which, for whatever reason, has just taken a long time to find adoption. I think because of generative AI, the focus is back on AI, whether traditional or some of the newer AI models. While it took seven or eight years for the internet and mobile apps to find a way to get monetized, that's not the case with AI. I think this will be monetized much quicker than what we've seen in the past.
But, when I linked those two together (Internet and AI), I was really linking the fact that AI is transformational. It's not a gadget or an app; it's truly a transformational technology that will change how we do things.
Sean Riley:
Could you give some general examples of where manufacturing companies have already used AI that might surprise people?
David McGraw:
There are some obvious ones, like predictive maintenance. That's a low-hanging fruit use case. I think it's one of the more commonly adopted use cases because it's simple for everyone to understand the goal. But by no means is it a tremendously easy use case to implement, depending on the amount of data you have readily available to you and what it will take to get the data.
The other one is quality control. Where you have vision inspection systems at different areas on your assembly line or throughout the manufacturing process. Some of those systems have been around for a long time.
There's supply chain optimization. And when I say supply chain optimization, there are so many ways to look at it. You could look at it from a logistics perspective, from demand forecasting to optimizing delivery routes to managing inventory levels. That’s a big, broad bucket of potential use cases.
I don't know of any company that uses those technologies better than Amazon does. Amazon runs millions of machine learning models behind the scenes to predict what Sean Riley will buy, and it makes sure that the warehouse closest to you has those products. So when you order it, you get it within 24 hours. From an Amazon perspective, AI has made a huge difference.
Another use case that's getting more popular is energy management. It involves trying to understand how and when to use energy best to keep some of the costs down while reducing the consumption of energy. There are all types of systems out there to help with this. How much predictability or machine learning is in some of those systems? I can't say for sure, but I do think that's going to be an area of focus in the future.
The last one I'll touch on is worker safety. I've worked on a few of these use cases in the past. This is a tough one, though, as there are a lot of benefits, but there are some legality concerns. There seems to be something taboo about monitoring people and how they work, even if it's to improve their workplace safety. There are other aspects of that, specifically around unions, too, because now you have yet another party you must satisfy. It’s not the easiest one.
Sean Riley:
With these examples and others in mind, how can embracing AI to optimize processes, increase efficiency, and bring manufacturing fully into the 21st century?
David McGraw:
There are a couple of use cases I've worked on. One of them was around process optimization and predictive analytics. You have an operator in a manufacturing facility who essentially controls the inputs, such as the speed at which the machine will run and some other parameters they can access. My observation has been that operators, even if they're told, “always keep the parameters at X, Y, and Z,” if they have been on these machines for a long time, are still likely to adjust it based on what they've seen in the past, what they're comfortable with, and what they've had the most success with.
Imagine a situation where I have data that I'm collecting on all the runs on this machine. My machine learning algorithms say for these parameters, set X, Y, and Z to show 1, 2, and 3, for example, which will predictably give you the best output. But it might not necessarily be based on output. There will be a control variable that you are trying to optimize for with AI. And whatever that variable is, your model would essentially predict what those parameters need to be set to. That's a good example. I have seen that in production. It works well, as far as improving throughput and quality. So, AI is a great use case to optimize processes.
There's also efficiency with Cobots. If you remember, when we started to come out of COVID, Cobots—which had been introduced long ago—started gaining popularity because manufacturing companies could not find workers. So they got these Cobots, which, essentially, it's a Cobot because it's collaborating with humans using AI. In the scenario that I described, when we were coming out of COVID, and we had all these resource issues, it was a really good plug and play solution for companies. It worked well, and humans were very much in the loop with AI.
When we think about AI, there are parts of its use that require humans to be involved; there are also some use cases where that is not as important. Process optimization is one that sits right dead in the middle. But with Cobots, clearly, there has to be a human in the loop.
The next thing I would talk about would be around quality control. That's a huge advantage with AI if you're looking at your OEE. Having the ability to foresee a potential issue, some anomaly on the widget you're packaging. Or is there something wrong? Not only does it save time, but when you have a vision system using AI, you can potentially get every one of the packages correct whereas a human just wouldn't be able to do that as efficiently. You're going to increase your quality and realize any defects quicker. And all that data that you collect, if you feed that back into machine learning models, there is that opportunity to improve the process.
So that first use case, I talked about, some of that data might feed in; maybe you have to change some of the parameters, maybe you're running too fast, and it's causing some quality issues. Or that data gets fed back to some of the product managers. And maybe there's a flaw in the package or product being packaged that needs to be addressed. There are many, many scenarios where that data can be very relevant, not just to improve quality, but to improve the process. What you start to see is as you collect the data from a vision system, from your Cobots, from predictive maintenance or process optimization, you bring it all together, and you start to have endless opportunities for use cases that you can go and solve.
Sean Riley:
Could you walk us through AI generated predictive maintenance and how AI benefits the user?
David McGraw:
That's a use case I know really well. The first thing you have to do is just be able to get data off of the machines. If you're starting your journey there and don’t have smart machines, you're going to add third-party sensors to start collecting that data, which will feed into the cloud or edge device. This is where your AI machine-learning models are going to run. In the ideal world, if there will be some failure, that prediction will give a day's notice or maybe four hours, at a minimum so that a person can act on it. It takes effort to train those machine learning models; you must have good data. And if you're trying to predict a specific type of failure, you're going to need that specific type of failure in your data set as well. So the models can learn different types of failures. Now, when it predicts, it might be smart enough to say not only is the machine going to fail, but it is also going to fail because of X, Y, or Z. If you can get your models to do that, where it tells you the X, Y, or Z it might be possible to then integrate with a generative AI that sits over top a bunch of your reference documentation, and it provides you the instruction to go fix it and the tools you'll need. That's a kind of Nirvana-type scenario, but it will be possible.
The big benefits are moving unplanned downtime to planned downtime and improving the safety of the operator, who would have to crawl all over the machine to find the cause of the failure.
To view the entire webinar, please download our on-demand recording here.