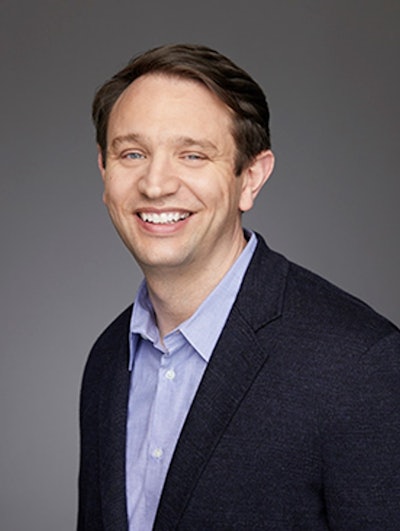
David McGraw is a seasoned professional in the field of Artificial Intelligence. As a Senior Director at Alvarez & Marsal in Miami, he specializes in applying Generative AI to enhance performance and create value across various business sectors, including manufacturing. At PMMI’s 2023 Annual Meeting, he presented “AI in Manufacturing Ops – How is your Company Using AI?” before sitting down with Sean Riley for an upcoming podcast episode of unPACKed with PMMI. The following is an excerpt of that podcast edited for content and clarity.
Sean Riley:
During your presentation at PMMI’s Annual Meeting, you presented three advantages of generative AI versus traditional AI. What do you feel is the most significant advantage?
David McGraw:
Yeah. So right now, the biggest advantage of generative AI is that tools like ChatGPT tools like Bard and Claude are available, and anyone can use them. It totally democratizes the use of AI. There are zero barriers to entry and zero cost to you to play with it and see what it can do. Generative AI, you can have it right now at no cost to you. Companies like Open AI and Google have done all the heavy lifting for you. These models exist today, and you can play with them right now. Nothing prevents you from doing it other than logging on to the machine and doing it.
Riley:
How does that run counter to traditional AI?
McGraw:
With traditional AI, many of the use cases I talked about on the plant floor are complex. You must figure out, "How will I start collecting data?”
Predictive maintenance use case for traditional AI
So now, you're already six months to two years from the beginning before you really are doing anything of actual value or interest despite the cost. Then, what are you going to do? You have to start writing the algorithms with your dataset. Then you start testing it and say, "Okay. I can get this level of accuracy in my predictions." What if that accuracy is low? Then, you must go back to the drawing board.
And it's very iterative. And because there's latency between getting the data and doing something with it, it can get costly. And the time-consuming aspect is the hard part because we've all been preconditioned. We want everything right now.
Riley:
AI is it's a touchy subject for some people. It's scary. They say, “It's going to take my job. It's going to replace me.” How do you eliminate some of the concerns people may have about risk, and how do you build trust with using AI?
McGraw:
That is a fantastic question. Until recently, the last 13 years of my career have generally been spent in manufacturing. It's always been hard for me because I've been someone who's been pushing AI, and the use of it and manufacturing is not always “bleeding edge” with new technologies. So, trust comes from multiple areas: trust in the results or trust in the insights. That'll always be very hard to build trust in because there will always be naysayers. And AI is not perfect; it'll never be perfect. I don't ever see a time when it will be 100%. Remember, it's just like us. We make mistakes; we have flaws. It's going to act very similar to us. So, there's that level of trust.
And what is so frustrating for me is when it comes to AI, and it's basically because of some of the things you mentioned, there's a fear of it. We hold its outputs at a higher level than the outputs of humans. It's wrong one or two times. But when a person is wrong twice or thrice, it's not a problem, "Humans make mistakes."
So, that's always been a frustrating piece for me.
Riley:
There's an expectation of perfection.
McGraw:
There's an expectation of perfection, but the same expectation doesn't exist with humans. There's also a different lens of trust that we can look at. And it's this trust of, "Will it take our jobs?"
As I mentioned during my presentation, the fear should be, if you have a set of people in your organization that use AI and a set of people in your organization that don't, the folks that use it will probably be around for the long term. The people who don't use AI won't. The analogy I use is early in my career, when I was a low-level programmer, I sat next to folks who were more experienced. I'd run into an issue and search on Google to see how I could resolve it.
I would find solutions, but the folks who weren't using the internet would laugh at me. And they're like, "That's dumb. Just look in the reference manual." What would take me minutes would take them hours. And what ultimately ended up happening is, in the span of three to five years, I was there, and they weren't. Whether or not they could find a job probably depended on whether they were willing to adapt and adopt the Internet.
This is even more transformational than access to the Internet because, on the Internet, you search, you get a lot of links, and you have to go through the links to find the correct answer. Here, it gives you the answer most of the time. Look at 50 years ago, everyone wasn’t interacting with a computer. Now, it's my phone is a computer.
Riley:
I don't feel that the internet was thought of as “scary” like AI is, for lack of a better word, even though it did have the potential to take people's jobs. But like you said, it was more people being willing to use a tool versus those not willing to use it that got left behind.
McGraw:
And I think it'll happen here. And look, AI will always have this bad taste in people's mouths because of all the movies and how AI has been presented.
It'll have challenges, but it'll also create new jobs. Other folks' jobs will change over time. And even at the firm I'm at now, one of the things we're talking about is, "Well, we have to get our consultants really good at prompt engineering."
So that's going to become a skillset.
Riley:
When do you think the government will step in from a regulatory standpoint on AI?
McGraw:
What's ultimately going to happen is the government will be slow to react until something significant happens. That's what scares me the most. I don't blame the government; AI is moving rapidly. But in Washington, it's challenging to get all the right people in a room together and agree on anything, much less, "What's the policy we should run around AI?"
Everyone's coming with their own agenda so it will be a struggle. The truth of the matter is, and the sad fact is, something bad will probably have to happen, and then regulations will be overshot, and it will potentially clip some of the innovation.
But we’ll see.